Sustainable Mining with Artificial Intelligence and Machine Learning
July 2024
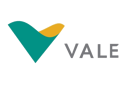
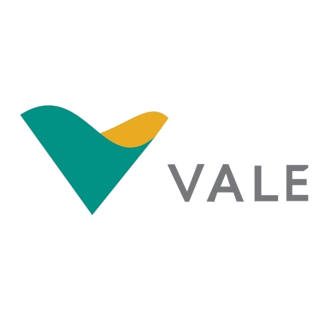
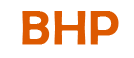
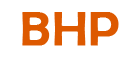
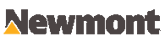
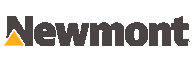

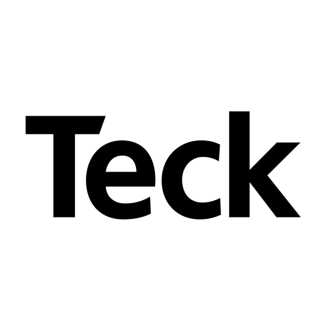
Rio Tinto : Optimizes operations by using AI and ML to automate data collection from trucks, conveyors, trains, and ships.
Vale : Established the Vale Artificial Intelligence Center to work on projects such as rail fracture prevention, train wheelset maintenance, mine asset management, fuel consumption reduction, pelletizing process optimization, and safety incident prevention.
BHP : Partners with Microsoft to use AI for optimizing copper production at Escondida and process area set point optimization.
Fortescue : Utilizes approximately 200 autonomous trucks, covering millions of kilometers and transporting large amounts of material.
Barrick : Implements machine learning at the mine’s hydroelectric power stations, reducing the need for backup diesel generation.
Goldcorp : Uses IBM Watson for big data analysis, aiding in the discovery of new mineral deposits.
Teck : Through the RACE21™ program, employs AI and ML algorithms for mine optimization, process improvements, integrated operations and maintenance, reducing health and safety risks, and enhancing sustainability.
AngloAmerican : Uses AI to simulate different processes and take preventive measures in actual operations.
The mining sector is undergoing a transformation with the integration of artificial intelligence (AI) and machine learning (ML) technologies. Leading mining companies worldwide are adopting these technologies to enhance operational efficiency, reduce costs, and minimize environmental impacts. Here are some examples of current applications: (*)
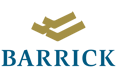
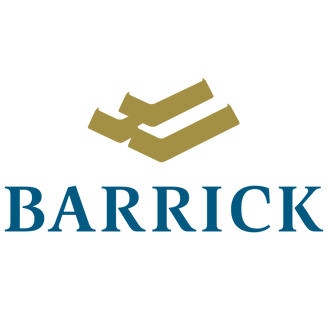
POTENTIAL AREAS FOR ARTIFICIAL INTELLIGENCE AND MACHINE LEARNING IN MINING
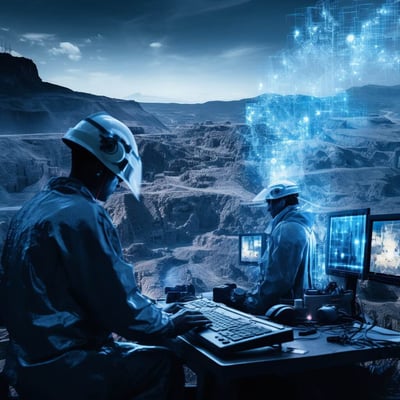
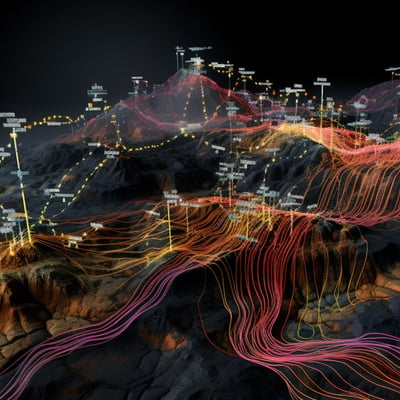
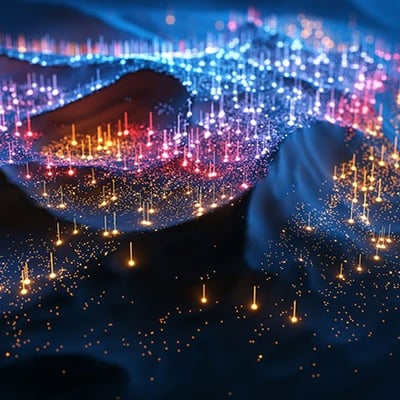
AI and ML have the potential to be applied more broadly in the mining sector, fundamentally transforming the industry over the next 10-15 years. Here are areas ripe for innovation and development:
Strategic Planning: Predicting future market demand and price movements, forecasting potential risks, and adverse scenarios.
Mine Planning: Accurately predicting mineral distribution and density to ensure the most efficient use of reserves.
Grade Assignment: Using artificial neural networks (ANN) to determine grade values with high accuracy and dynamically updating these values with continuous data flow.
Cost Accounting: Accurately forecasting project costs and making long-term predictions of revenues and expenses.
Process Optimization: Automating production processes and continuously monitoring and optimizing product quality and efficiency parameters in real-time.
Quantum Computing for Learning Algorithms: Accelerating traditional machine learning algorithms with quantum computing to solve more complex problems with high processing power.
Environmental Monitoring and Management: Continuously monitoring water, air, and soil quality at mining sites, minimizing environmental impacts, and developing strategies to enhance environmental sustainability.
Energy Management and Optimization: Improving energy efficiency and reducing energy costs by integrating renewable energy sources into mining operations.
Maintenance and Repair Management: Predicting equipment failures in advance, optimizing maintenance schedules, and performing maintenance and repairs remotely.
Worker Safety and Health: Continuously monitoring worker health conditions, minimizing health risks, and ensuring worker safety through smart sensors and monitoring systems.
Geological Exploration and Mapping: Using AI-supported analyses to discover new mineral deposits and creating three-dimensional maps of mining sites.
Autonomous Mines: Fully automated mining sites operated by AI, with no need for human intervention, where operational processes are completely managed by AI.
Smart Sensors and IoT: Connecting all mining equipment with the Internet of Things (IoT) and using advanced sensors to collect and analyze environmental and operational data.
Biomining:
- Bioleaching; A method where bacteria and fungi dissolve metal sulfides, making the metals more accessible. This method is particularly used for extracting valuable metals like copper, gold, and uranium.
- Bioremediation: Using microorganisms to clean contaminated mining sites by breaking down harmful substances or converting them into less harmful forms.Genetically Modified Organisms (GMOs) and Genomics:
- Enhanced Bioleaching Microbes: Genetically modifying microorganisms to increase the solubility of metals. These modified microbes dissolve metal sulfides more efficiently, speeding up the metal extraction process. This method is especially advantageous for low-grade ores.
- Phytomining: Using plants to extract metals from the soil through their root systems. These plants absorb metals from the soil and accumulate them in their leaves. Genetically modified plants are developed to increase metal accumulation. This method is used for extracting valuable metals from low-grade soils.
EQUALITY IN ACCESS TO TECHNOLOGY
The accessibility of technology between developed and developing countries has never been as equitable as it is today. However, industries in developing countries that fail to adapt these technologies face an inevitable struggle. Therefore, the widespread adoption of AI and machine learning technologies in the mining sector is crucial for a sustainable future. This translation maintains a professional tone and incorporates mining jargon to ensure clarity and effectiveness. Let me know if there are any adjustments you'd like to make!
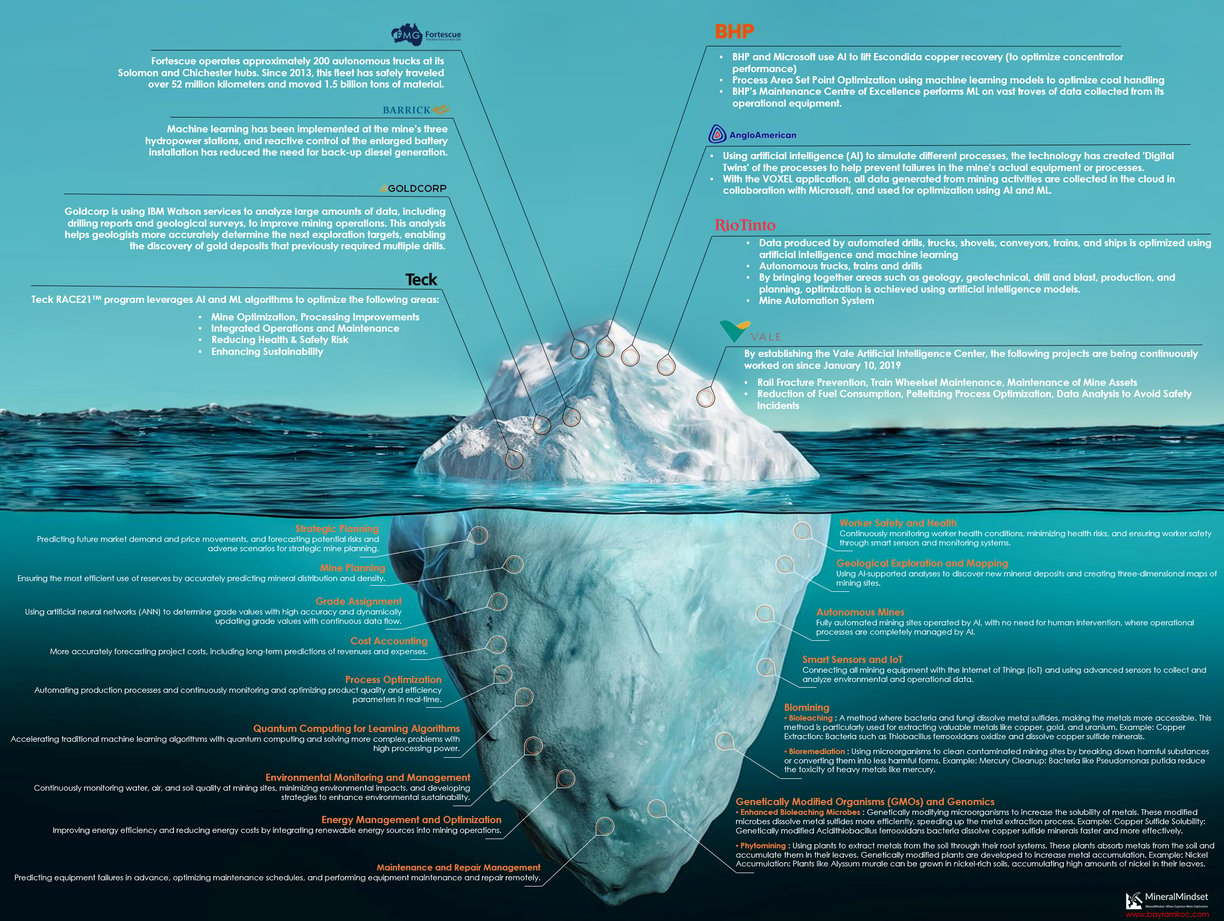
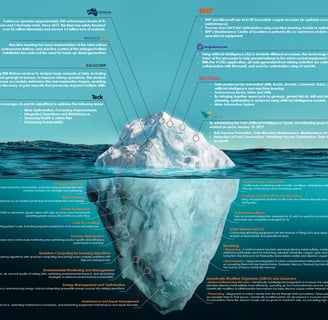
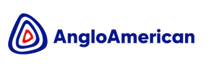
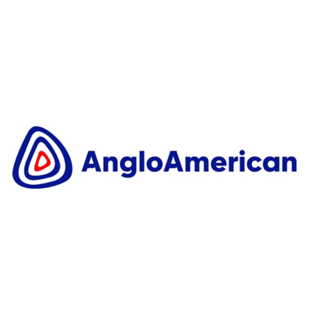
(*) The information provided about the companies is based on company statements and press releases.